From Markers to Machine Learning: Transforming Cell Type Annotation with GPT-4
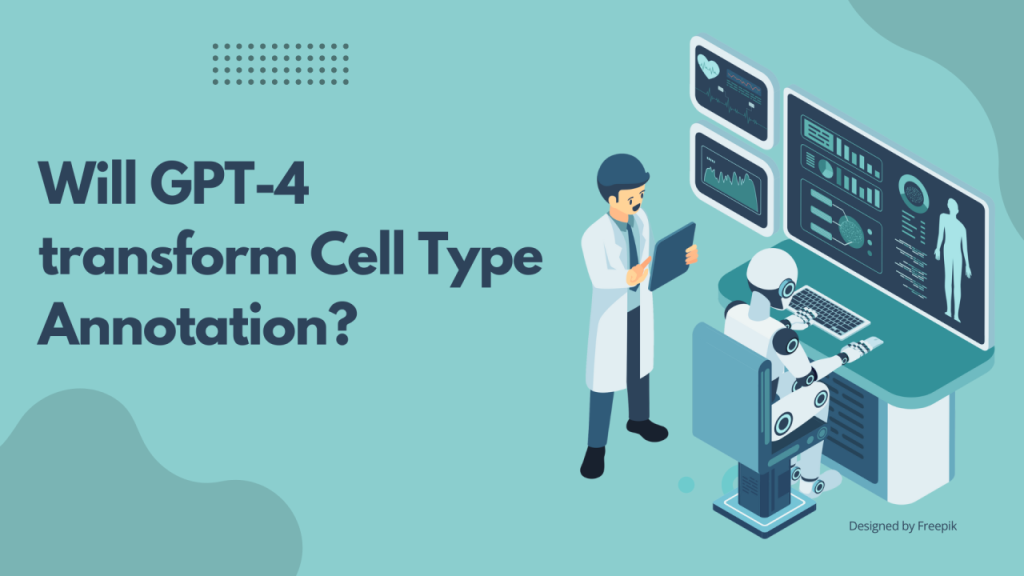
Introduction to Single-cell RNA Sequencing and Cell Type Annotation
In recent years, Single-cell RNA sequencing (scRNA-seq) has emerged as a powerful tool for advancing biological research by enabling the exploration of cellular heterogeneity at high resolution. One of the key steps in scRNA-seq analysis is cell type annotation, where cells are assigned biological identities based on their gene expression profiles. Accurate cell type annotation is essential for various downstream applications, such as understanding cellular functions, interactions, and pathways involved in complex biological processes.
Traditionally, cell type annotation involved looking at the gene expression patterns in different cell groups or clusters and comparing them to known marker genes associated with specific cell types. Marker genes are genes that are highly expressed in certain cell types, which makes them useful for identifying cell types of interest. This process, however, was time consuming and heavily dependent on prior knowledge of marker genes. Recent advancements in automated methods have significantly improved the efficiency and accessibility of cell type annotation. In this overview, we explore the leading tools and methodologies in this evolving field, alongside promising new developments in AI-based approaches.
GPT-4’s Role in Automating cell type Annotation
One of the promising approaches to automated cell type annotation is the use of GPT-4, a large language model that streamlines this process. Previously, experts manually compared highly expressed genes in cell clusters to known marker genes, which was labour-intensive. GPT-4 has simplified this task, as demonstrated in a study by Hou et al. 2024,where GPT-4’s performance was compared with traditional methods like SingleR, ScType, and CellMarker2.0. The model achieved comparable or superior accuracy, aligning with manual expert annotations in over 75% of cases.
GPT-4 is especially effective at identifying immune cell types and distinguishing between normal and malignant cells. Its ease of integration with existing workflows, such as Seurat, and low cost (approximately $0.10 per query) make it a valuable tool for labs seeking to optimise cell type annotation.
Comprehensive approaches to Automated Cell Type Annotation
In addition to GPT-4, other approaches to automated annotation can be categorised into three primary methods: marker gene-based annotation, correlation-based methods, and supervised classification.
- Marker Gene-based Annotation: This method utilises databases such as CellMarker and PanglaoDB, which contain gene markers for specific cell types. Tools like scCATCH and SCSA compare gene expression profiles with these markers to assign cell types. While fast and effective for well-characterised cell types, it may struggle with novel or poorly represented cells.
- Correlation-driven Annotation: Tools such as SingleR and scmap employ correlation-based techniques, comparing gene expression profiles from query datasets to reference datasets. By calculating correlations, they assign the most similar cell type. This method is flexible and useful for data lacking clear markers, but it can be computationally intensive for large datasets.
- Supervised Classification: Machine learning-based methods, including Garnett and scClassify, use supervised models trained on labelled datasets to predict cell types in new datasets. These models offer high accuracy and can handle complex cell type hierarchies. However, they require high-quality labelled training data, which can be a limiting factor.
Comparative analysis of different approaches
Each of these methods has unique strengths and limitations: Marker gene-based approaches are rapid but may not handle novel or ambiguous cell types well. Correlation-driven methods are adaptable but may be computationally expensive. Supervised classification methods provide high precision but depend heavily on the availability of well-annotated training data.
While GPT-4 and other automated methods represent significant advancements, challenges remain. AI models can produce errors, especially with noisy data or poorly defined gene sets. Thus, human validation remains essential to ensure the accuracy of automated annotations.
Conclusion: The path forward
Automated cell type annotation is progressing rapidly, with tools like GPT-4 and other emerging technologies offering potential for enhancing how we analyze scRNA-seq data. As these technologies continue to evolve, there is a growing opportunity to assess their impact on improving accuracy and efficiency. While promising, further exploration is needed to determine whether LLMs like GPT-4 can surpass existing automated methods in improving cell type annotation accuracy and furthering biological research.
Authored by: Deepshikha Singh
References
- Achiam, J., Adler, S., Agarwal, S., Ahmad, L., Akkaya, I., Aleman, F. L., … & McGrew, B. (2023). Gpt-4 technical report. arXiv preprint arXiv:2303.08774.
- Hou, W., & Ji, Z. (2024). A systematic evaluation of large language models for generating programming code. arXiv preprint arXiv:2403.00894.
- Zhang, X., Lan, Y., Xu, J., Quan, F., Zhao, E., Deng, C., … & Xiao, Y. (2019). CellMarker: a manually curated resource of cell markers in human and mouse. Nucleic acids research, 47(D1), D721-D728.
- Franzén, O., Gan, L. M., & Björkegren, J. L. (2019). PanglaoDB: a web server for exploration of mouse and human single-cell RNA sequencing data. Database, 2019, baz046.
- Shao, X., Liao, J., Lu, X., Xue, R., Ai, N., & Fan, X. (2020). scCATCH: automatic annotation on cell types of clusters from single-cell RNA sequencing data. Iscience, 23(3).
- Cao, Y., Wang, X., & Peng, G. (2020). SCSA: a cell type annotation tool for single-cell RNA-seq data. Frontiers in genetics, 11, 490.
- Liu, H., Harris, A., Jenkins-Lord, B., Dorsey, T. H., Makokha, F., Sayed, S., … & Ambs, S. (2024). Abstract LB240: Cell type annotation using singleR with custom reference for single-nucleus multiome data derived from frozen human breast tumors. Cancer Research, 84(7_Supplement), LB240-LB240.
- Kiselev, V. Y., Yiu, A., & Hemberg, M. (2018). scmap: projection of single-cell RNA-seq data across data sets. Nature methods, 15(5), 359-362.
- Pliner, H. A., Shendure, J., & Trapnell, C. (2019). Supervised classification enables rapid annotation of cell atlases. Nature methods, 16(10), 983-986.
- Lin, Y., Cao, Y., Kim, H. J., Salim, A., Speed, T. P., Lin, D. M., … & Yang, J. Y. H. (2020). scClassify: sample size estimation and multiscale classification of cells using single and multiple reference. Molecular systems biology, 16(6), e9389.